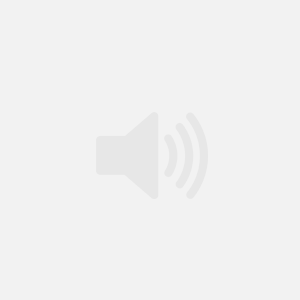
On this “Speaking of Bitcoin” episode, join hosts Adam B. Levine, Stephanie Murphy, Jonathan Mohan and special guest Martin Rerak, creator of AllYourFeeds.com, for a look at how “AI curation” is being used to figure out what’s useful information and what’s just fluff.
This episode is sponsored by Crypto.com, Nexo.io and Elliptic
Hundreds of tabs
In the early days of Bitcoin, there were just a few places you might go to read news and stay informed, but over the years things have changed dramatically. Today there are thousands of projects and hundreds of articles written each day. And that’s assuming you ignore the wilds of YouTube or the depths of crypto Twitter.
There were days I was waking up to a hundred tabs that I was basically just reloading from the prior day… You know, looking at Slack, Telegram, Twitter accounts, Discord, Reddit and dozens of publications online […] It was very easy to point somebody in the [right] direction if they’re saying, “Where can I buy cryptocurrency?” But if they were saying, “Is there a use case here for traceability?” or “What do you think I should invest in?” or “How is this project developing?” that becomes a lot more loaded and challenging… – Martin Rerak
See also: What Is GPT-3 and Should We Be Terrified?
In this episode, we discuss the crypto-media landscape, AI training, the challenges around bias and un-biasing practices, potential impacts of the natural-language-generating algorithm known as GPT-3 and more.
Biased AI
While unsettling on the surface, the idea of bias within an AI is not as controversial as you might imagine – it’s almost required. As humans, we each have our own experiences and preferences which shape our viewpoint and our biases. Modern artificial intelligence consumes “training material” curated by humans to learn what’s right or wrong for its particular task. Once trained, AI can help us with those tasks and is at its most useful when it’s “instincts” match whomever it is working on behalf of.
Of course whether bias is good or bad depends a lot of your priorities. When Google trained an AI to help with hiring, the data around past and current employees led it to believe that an ideal “Google engineer” wouldn’t have a woman’s college on their academic transcript. For Google, their past records did not match their future ambitions and so bias was a problem.
But personally, I’ve developed patent-pending AI technology that assists with audio editing, and here the idea of bias is critical. There is no objective standard of what sounds best, only personal preferences. For an AI to assist an audio editor, it must be in tune with those preferences and be able to make decisions that are objectively correct for the person it is assisting.
This is much the same with AI assisted news curation. We all have our own preferences, interests and biases which help us decide what we do or don’t care about. On today’s show we dig into this fascinating topic where one size rarely fits all and the future is wide open.
—
Send in a voice message: https://anchor.fm/originalltb/message